Power of Pandas¶
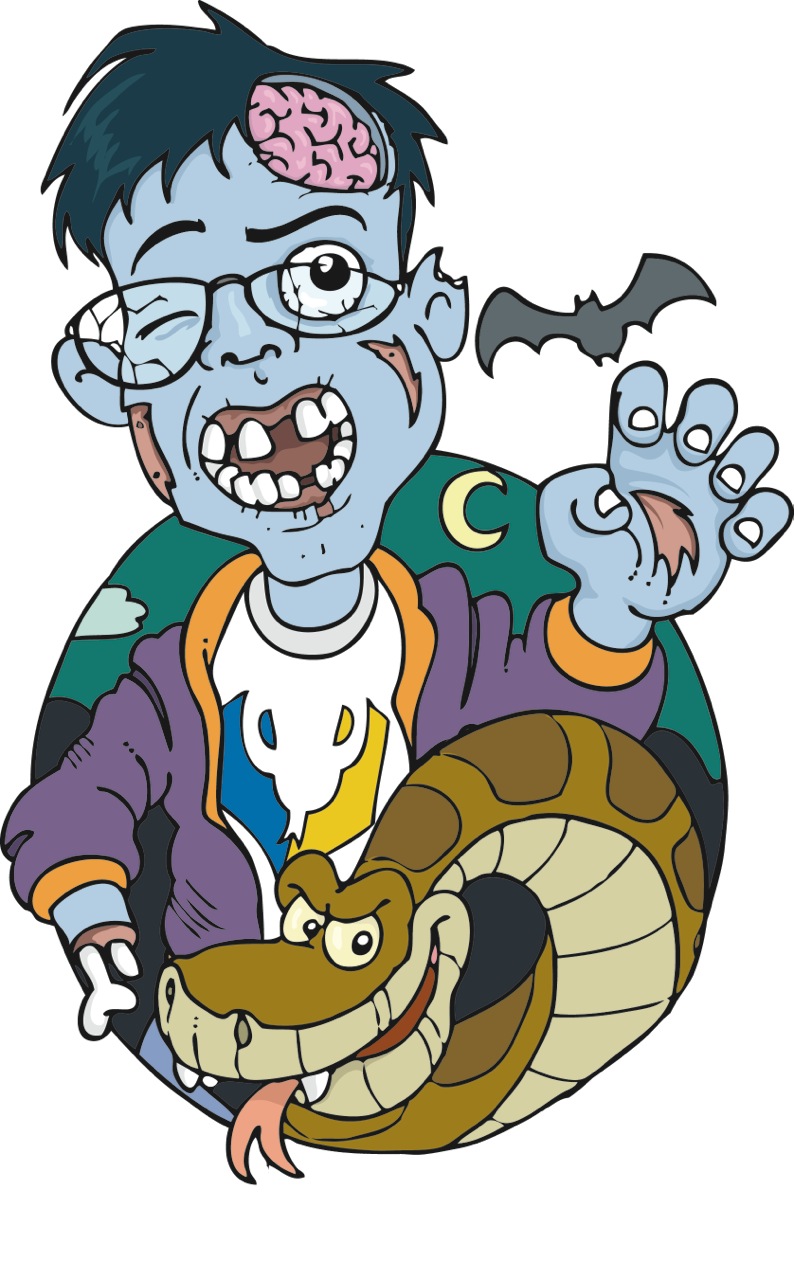
Introduction¶
This lecture is on one of the most powerful tools in the Python ecosystem for data analysis - Pandas. In this lecture, we’ll embark on a journey to explore the ins and outs of Pandas, understanding its capabilities in handling, manipulating, and analyzing data effectively.
Understanding Pandas Basics¶
Pandas, built on top of NumPy, provides data structures and functions to work with structured data.
Key components: Series (1-dimensional labeled array) and DataFrame (2-dimensional labeled data structure).
Importing Pandas and loading data:
import pandas as pd
andpd.read_csv()
.
Exploratory Data Analysis (EDA) with Pandas¶
Checking data dimensions:
df.shape
.Getting summary statistics:
df.describe()
.Examining data types and missing values:
df.info()
.
Data Cleaning and Transformation¶
Renaming columns for clarity:
df.rename(columns={'old_name': 'new_name'}, inplace=True)
.Handling missing data:
df.dropna()
,df.fillna()
.Data type conversion:
df.astype()
.
Data Manipulation and Aggregation¶
Selecting columns and rows:
df['column_name']
,df.loc[]
,df.iloc[]
.Filtering data:
df.query()
.Grouping and aggregating data:
df.groupby().agg()
.
Data Visualization with Pandas¶
Utilizing Matplotlib and Seaborn integration for visualizations.
Basic plots:
df.plot()
.Bar plots, histograms, box plots:
df.plot(kind='bar')
,df.plot(kind='hist')
,df.plot(kind='box')
.
Advanced Data Analysis Techniques¶
Time series analysis: Handling datetime data with Pandas.
Merging and joining datasets:
pd.merge()
,pd.concat()
.Handling duplicates:
df.drop_duplicates()
.
Real-world Applications and Case Studies¶
Analyzing healthcare data: Exploring patient wait times, service distribution, and geographical trends.
Financial data analysis: Stock market analysis, portfolio management.
Social media data analysis: Sentiment analysis, trend detection.
Best Practices and Performance Optimization¶
Efficient data loading and storage: Utilizing chunking, optimizing data types.
Vectorized operations: Leveraging Pandas’ vectorized operations for faster computations.
Memory management: Reducing memory usage for large datasets.
Example¶
Here is something you can develop using panda. This example uses the data of ice cream products from Beijing. The data is read from a CSV file and then visualized using matplotlib. The user can select a flavour of ice cream and the graph will display the rating of the selected flavour.
import js
import pandas as pd
import matplotlib.pyplot as plt
from pyodide.http import open_url
from pyodide.ffi import create_proxy
url = (
"https://raw.githubusercontent.com/Cheukting/pyscript-ice-cream/main/bj-products.csv"
)
ice_data = pd.read_csv(open_url(url))
current_selected = []
flavour_elements = js.document.getElementsByName("flavour")
def plot(data):
plt.rcParams["figure.figsize"] = (22,20)
fig, ax = plt.subplots()
bars = ax.barh(data["name"], data["rating"], height=0.7)
ax.bar_label(bars)
plt.title("Rating of ice cream flavours of your choice")
display(fig, target="graph-area", append=False)
def select_flavour(event):
for ele in flavour_elements:
if ele.checked:
current_selected = ele.value
break
if current_selected == "ALL":
plot(ice_data)
else:
filter = ice_data.apply(lambda x: ele.value in x["ingredients"], axis=1)
plot(ice_data[filter])
ele_proxy = create_proxy(select_flavour)
for ele in flavour_elements:
if ele.value == "ALL":
ele.checked = True
current_selected = ele.value
ele.addEventListener("change", ele_proxy)
plot(ice_data)